Unleash the Power of Multi-Modal Data: Pioneering Precision Medicine
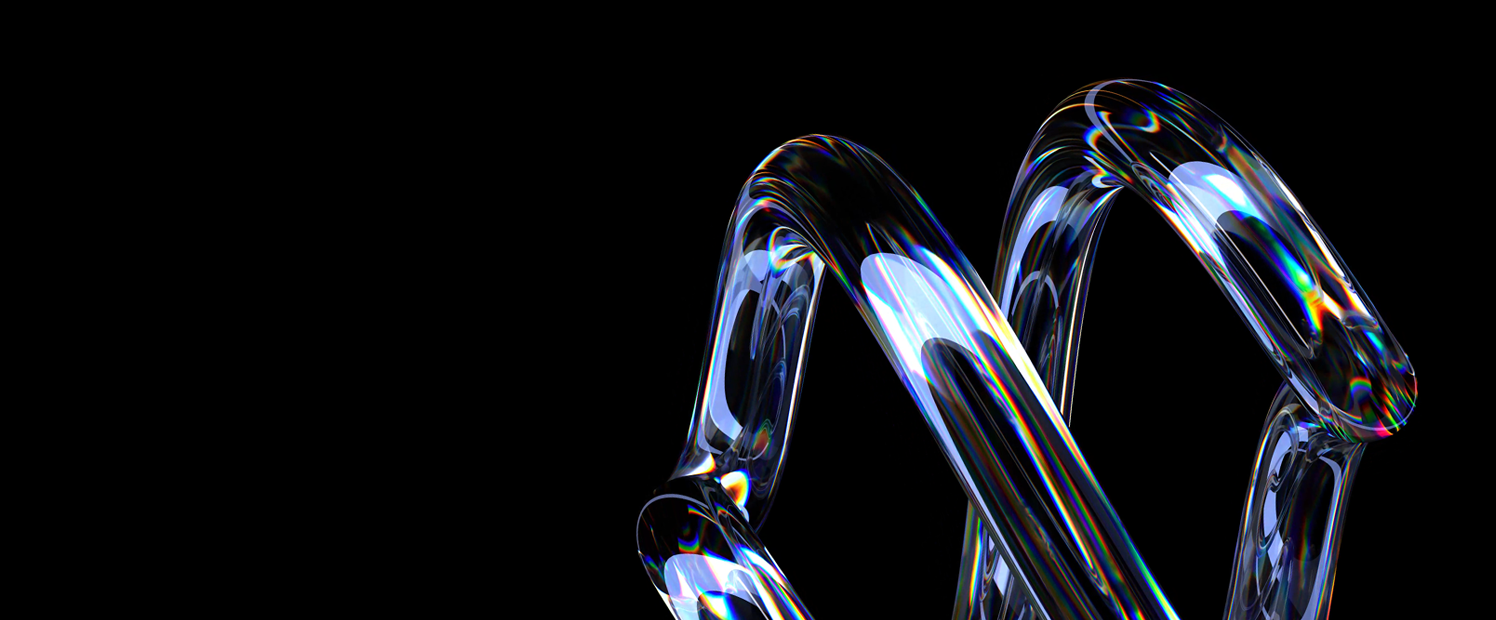
In recent years, precision medicine has witnessed remarkable progress, thanks to multi-omics and multi-modal data integration.[1,2] This holds immense potential for advancing healthcare in various domains, such as risk target identification, trial design, diagnostic analytics, population health analysis, lab automation, and clinical decision support[3,4,5]. Whether you are part of industry, government, or academia, leveraging multi-omics and multi-modal data can revolutionize how we translate data into actionable strategies for improving patient outcomes. In this blog post, we will explore the transformative power of these cutting-edge technologies and their contributions to advancing healthcare.
The Promise of Multi-Omics and Multi-Modal Data:
Multi-omics analyzes different molecular data types, including genomics, transcriptomics, proteomics, metabolomics, and epigenomics. By integrating these diverse datasets, researchers gain a more comprehensive understanding of disease mechanisms, uncover hidden patterns, and identify potential therapeutic targets[6]. Furthermore, combining multi-omics data with clinical, imaging, and environmental data in a multi-modal approach provides a holistic view of a patient’s health, facilitating precision medicine.
Advancing Science through Collaborative Efforts:
Velsera, a leading platform in precision medicine, has been at the forefront of facilitating collaborative efforts in this field. Working closely with esteemed partners such as the National Cancer Institute (NCI), the National Health Lung and Blood Institute (NHLBI), and pediatric health institutions, Velsera’s Science department has achieved significant milestones in priority areas identified by these organizations.
Consortia, research groups, individual researchers, and scientific trainees have embraced Velsera’s cutting-edge platforms, like the Cancer Genomics Cloud [7], BioData Catalyst[8], and CAVATICA[9], to conduct multi-omics and multi-modal research [10-12]. These collaborative platforms provide powerful computational tools, secure data-sharing capabilities, and streamlined workflows, enabling researchers to leverage vast amounts of data efficiently and effectively. By utilizing these resources, scientists have generated groundbreaking insights, accelerating scientific progress and improving patient outcomes [13-15].
Implications for Healthcare:
The application of multi-omics and multi-modal data has far-reaching implications for healthcare. Let’s explore some of the critical areas where these technologies have already made a significant impact:
Risk Stratification: By integrating genomic, clinical, and lifestyle data, researchers can develop models that accurately predict an individual’s risk of developing specific diseases. This information enables targeted preventive interventions and personalized risk management strategies.
Diagnosis and Prognosis: Multi-modal data analysis allows for more precise and early detection of diseases, including subtypes. Integrating molecular, imaging, and clinical data enhances diagnostic accuracy, enabling healthcare professionals to tailor treatment plans to individual patients. Additionally, longitudinal data integration facilitates the prediction of disease progression and prognosis, enabling timely interventions.
Treatment Selection and Response Monitoring: Multi-omics and multi-modal data enable the identification of biomarkers that predict treatment response, allowing for personalized therapeutic interventions. Furthermore, real-time monitoring of treatment response using multi-modal data helps optimize treatment strategies and minimize adverse effects.
Drug Discovery and Development: Leveraging multi-omics data, researchers can uncover novel drug targets and develop targeted therapies. Integrating multi-modal data provides valuable insights into drug safety, efficacy, and individual patient response, paving the way for precision medicine approaches in drug development.
Conclusion:
The era of precision medicine has arrived, propelled by integrating multi-omics and multi-modal data. Collaborative efforts among industry, government, and academia, supported by platforms such as Velsera’s Science, have demonstrated the transformative potential of these technologies. We can unlock new insights, refine healthcare strategies, and ultimately improve patient outcomes by harnessing the power of multi-omics and multi-modal data.
Want to learn more?
Are you interested in learning more about the power of multi-omics and multi-modal data? Watch our recent webinar, “Ushering a New Era of Precision Medicine with Multi-Modal Data,” on demand today. During this session we discussed the challenges and opportunities of working with multiple data types and how they can be integrated to gain a complete picture of biological systems.
###REFERENCES
1. Hasin Y, Seldin M, Lusis A. Multi-omics approaches to disease. Genome Biol. 2017 May 5;18(1):83. doi: 10.1186/s13059-017-1215-1. PMID: 28476144; PMCID: PMC5418815.
2. Olivier M, Asmis R, Hawkins GA, Howard TD, Cox LA. The Need for Multi-Omics Biomarker Signatures in Precision Medicine. Int J Mol Sci. 2019 Sep 26;20(19):4781. doi: 10.3390/ijms20194781. PMID: 31561483; PMCID: PMC6801754.
3. Di Sario G, Rossella V, Famulari ES, Maurizio A, Lazarevic D, Giannese F, Felici C. Enhancing clinical potential of liquid biopsy through a multi-omic approach: A systematic review. Front Genet. 2023 Apr 3;14:1152470. doi: 10.3389/fgene.2023.1152470. PMID: 37077538; PMCID: PMC10109350.
4. Li CX, Wheelock CE, Sköld CM, Wheelock ÅM. Integration of multi-omics datasets enables molecular classification of COPD. Eur Respir J. 2018 May 10;51(5):1701930. doi: 10.1183/13993003.01930-2017. PMID: 29545283.
5. Ayton SG, Pavlicova M, Robles-Espinoza CD, Tamez Peña JG, Treviño V. Multiomics subtyping for clinically prognostic cancer subtypes and personalized therapy: A systematic review and meta-analysis. Genet Med. 2022 Jan;24(1):15-25. doi: 10.1016/j.gim.2021.09.006. Epub 2021 Nov 30. PMID: 34906494.
6. Nativio R, Lan Y, Donahue G, Sidoli S, Berson A, Srinivasan AR, Shcherbakova O, Amlie-Wolf A, Nie J, Cui X, He C, Wang LS, Garcia BA, Trojanowski JQ, Bonini NM, Berger SL. An integrated multi-omics approach identifies epigenetic alterations associated with Alzheimer’s disease. Nat Genet. 2020 Oct;52(10):1024-1035. doi: 10.1038/s41588-020-0696-0. Epub 2020 Sep 28. Erratum in: Nat Genet. 2020 Nov;52(11):1266. PMID: 32989324; PMCID: PMC8098004.
7. Lau JW, et al. The Cancer Genomics Cloud: Collaborative, Reproducible, and Democratized-A New Paradigm in Large-Scale Computational Research. Cancer Res. 2017 Nov 1;77(21):e3-e6. doi: 10.1158/0008-5472.CAN-17-0387. Erratum in: Cancer Res. 2018 Sep 1;78(17):5179. PMID: 29092927; PMCID: PMC5832960.
8. Ahalt S, et. al. Building a collaborative cloud platform to accelerate heart, lung, blood, and sleep research. J Am Med Inform Assoc. 2023 May 16:ocad048. doi: 10.1093/jamia/ocad048. Epub ahead of print. PMID: 37192819.
9. Heath A, et al. TMOD-19. GABRIELLA MILLER KIDS FIRST DATA RESOURCE CENTER: LARGE-SCALE HARMONIZED CLINICAL AND GENOMIC DATA PLATFORM TO SUPPORT CHILDHOOD CANCER AND STRUCTURAL BIRTH DEFECT RESEARCH. Neuro Oncol. 2019 Apr;21(Suppl 2):ii125. doi: 10.1093/neuonc/noz036.256. Epub 2019 Apr 23. PMCID: PMC6477273.
10. Koc S, Lloyd MW, Grover JW, Xiao N, Seepo S, Subramanian SL, Ray M, Frech C, DiGiovanna J, Webster P, Neuhauser S, Srivastava A, Woo XY, Sanderson BJ, White B, Lott P, Dobrolecki LE, Dowst H; PDXNet Consortium; Evrard YA, Wallace TA, Moscow JA, Doroshow JH, Mitsiades N, Kaochar S, Pan CX, Chen MS, Carvajal-Carmona L, Welm AL, Welm BE, Lewis MT, Govindan R, Ding L, Li S, Herlyn M, Davies MA, Roth J, Meric-Bernstam F, Robinson PN, Bult CJ, Davis-Dusenbery B, Dean DA 2nd, Chuang JH. PDXNet portal: patient-derived Xenograft model, data, workflow and tool discovery. NAR Cancer. 2022 Apr 22;4(2):zcac014. doi: 10.1093/narcan/zcac014. PMID: 35475145; PMCID: PMC9026194.
11. Zhuang Y, Hobbs BD, Hersh CP, Kechris K. Identifying miRNA-mRNA Networks Associated With COPD Phenotypes. Front Genet. 2021 Oct 28;12:748356. doi: 10.3389/fgene.2021.748356. PMID: 34777474; PMCID: PMC8581181.
12. Evrard YA, Srivastava A, Randjelovic J, Doroshow JH, Dean DA 2nd, Morris JS, Chuang JH; NCI PDXNet Consortium. Systematic Establishment of Robustness and Standards in Patient-Derived Xenograft Experiments and Analysis. Cancer Res. 2020 Jun 1;80(11):2286-2297. doi: 10.1158/0008-5472.CAN-19-3101. Epub 2020 Mar 9. PMID: 32152150; PMCID: PMC7272270.
13. Ma J, Joehanes R, Liu C, Keshawarz A, Hwang SJ, Bui H, Tejada B, Sooda M, Munson PJ, Demirkale CY, Courchesne P, Heard-Costa NL, Pitsillides AN, Feolo M, Sharopova N, Vasan RS, Huan T, Levy D. Elucidating the genetic architecture of DNA methylation to identify promising molecular mechanisms of disease. Sci Rep. 2022 Nov 15;12:19564. doi: 10.1038/s41598-022-24100-0. PMCID: PMC9664436.
14. Fuentes Bolanos NA, Padhye B, Daley M, Hunter J, Hetherington K, Warby M, Courtney E, Kirk J, Josephi-Taylor S, Chen Y, Alvaro F, Barlow-Stewart K, Wong-Erasmus M, Barahona P, Ajuyah P, Altekoester AK, Tyrrell VJ, Lau
LMS, Wakefield C, Sylvester D, Tucker K, Pinese M, Dalla Pozza L, O’Brien TA. Protocol for a comprehensive prospective cohort study of trio-based whole-genome sequencing for underlying cancer predisposition in paediatric and adolescent patients newly diagnosed with cancer: the PREDICT study. BMJ Open. 2023 May 30;13(5):e070082. doi: 10.1136/bmjopen-2022-070082. PMID: 37253493; PMCID: PMC10254808.
15. Chatrath A, Kiran M, Kumar P, Ratan A, Dutta A. The Germline Variants rs61757955 and rs34988193 Are Predictive of Survival in Lower Grade Glioma Patients. Mol Cancer Res. 2019 May;17(5):1075-1086. doi: 10.1158/1541-7786.MCR-18-0996. Epub 2019 Jan 16. PMID: 30651372; PMCID: PMC6497557.